What is Human in the Loop?
Understanding the Basics
By Christina Catenacci
Oct 18, 2024
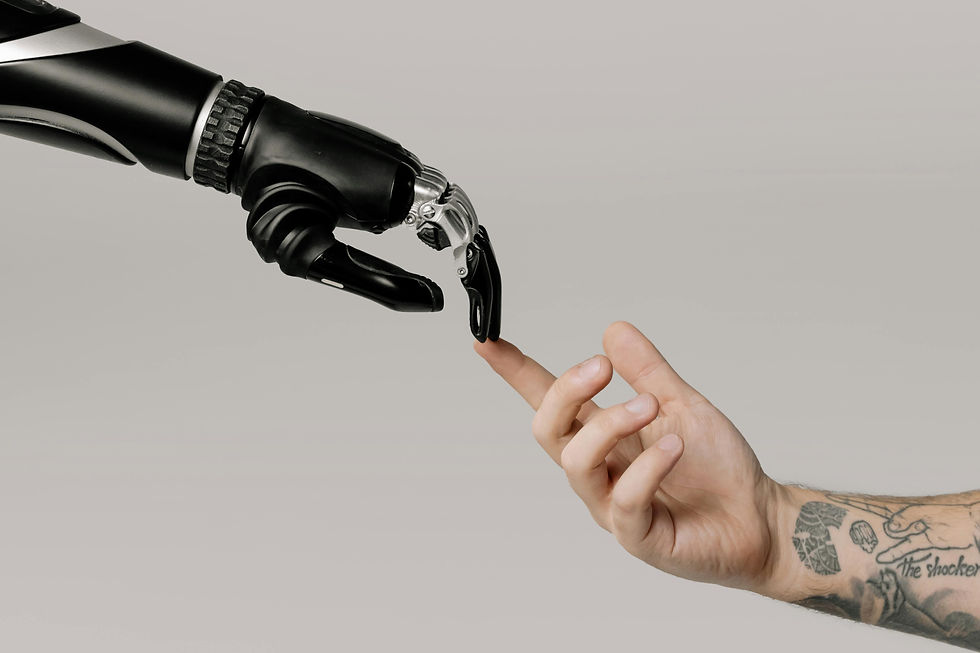
Key points
A human-in-the-Loop approach is the selective inclusion of human participation in the automation process
There are some pros to using this approach, including increased transparency, an injection of human values and judgment, less pressure for algorithms to be perfect, and more powerful human-machine interactive systems
There are also some cons, like Google’s overcorrection with Gemini, leading to historical inaccuracies
Humans-in-the-Loop - What Does That Mean?
A philosopher at Stanford University asked a computer musician if we will ever have robot musicians, and the musician was doubtful that an AI system would be able to capture the subtle human qualities of music, including conveying meaning during a performance.
When we think about Humans-in-the-Loop, we may wonder exactly what this means. Simply put, when we design with a Human-in-the-Loop approach, we can imagine it as the selective inclusion of human participation in the automation process.
More specifically, this could manifest as a process that harnesses the efficiency of intelligent automation while remaining open to human feedback, all while retaining a greater sense of meaning.
If we picture an AI system on one end, and a human on the other, we can think of AI as a tool in the centre: where the AI system asks for intermediate feedback, the human would be right there (in the loop) providing some minor tweaks to refine the instruction and giving additional information to help the AI system be more on track with what the human wants to see as a final output. There would be arrows that go from the human to the machine and from the machine to the human. By designing this way, we have a Human-in-the-Loop interactive AI system.
We can also view this type of design as a way to augment the human—serving as a tool, not a replacement.
So, if we take the example of computer music, we could have a situation where the AI system starts creating a piece, and then the human musician could provide some “notes” (pun intended) for the AI system that adds meaning and ultimately begins creating a real-time, interactive machine learning system.
What exactly would the human do? The human musician would be there to iteratively, efficiently, and incrementally train tools by example, continually refining the system. As a recording engineer and producer myself, I like and appreciate the idea of AI being able to take recorded songs and separate it into its individual stems or tracks, like keys, vocals, guitars, bass and drums. This is where the creativity begins…
What are the pros and cons of this approach?
While there are several benefits of using this approach, here are a few:
There would be considerable gains in transparency: when humans and machines collaborate, there is less of a black box when it comes to what the AI is doing to get the result
There would be a greater chance of incorporating human judgment: human values and preferences would be baked into the decision-making process so that they are reflected in the ultimate output
There would be less pressure to build the perfect algorithm: since the human would be providing guidance, the AI system only needs to make meaningful progress to the next interaction point—the human could show a fermata symbol, so the system pauses and relaxes (pun intended)
There could be more powerful systems: compared to fully automated or fully human manual systems, Human-in-the-Loop design strategies often lead to even better results
Accordingly, it would be highly advantageous to think about AI systems as tools that humans use to collaborate with machines and create a great result. It is thus important to value human agency and enhanced human capability. That is, when humans fine-tune (pun intended) a computer music piece in collaboration with an AI system, that is where the magic begins.
On the other hand, there could be cons to using this approach. Let us take the example of Google’s Bard (later renamed Gemini)—a mistake that cost the company dearly because it lost billions of dollars in shares and was quite an embarrassment.
What happened? Gemini, Google’s new AI chatbot at the time, began answering queries incorrectly. It is possible that that Google was rushing to catch up to OpenAI’s new ChatGPT at the time. Apparently, there were issues with errors, skewed results, and plagiarism.
The one issue that applies here is skewed results. In fact, Google apologized for “missing the mark” after Gemini generated racially diverse Nazis. Google was aware that GenAI had a history of amplifying racial and gender stereotypes, so it tried to fix those problems through Human-in-the-Loop design tactics, which went too far woke.
In another example, Google generated a result following a request for “a US senator from the 1800s” by providing an image of Black and Native American women (the first female senator, a white woman, served in 1922).
Google stated that it was working on fixing this issue, but the historically inaccurate picture will always be burned in our minds and make us question the accuracy of AI results. While it is being fixed, certain images will not be generated.
We see then, what can happen when humans do not do a good job of portraying history correctly and in a balanced manner. While these examples are obvious, one may wonder what will happen when there are only minor issues with diverse representation or inaccuracies due to human preferences…
Another con is that Humans-in-the-Loop may not know how to eradicate systemic bias in training data. That is, some biases could be baked right into the training data. We may question: who is identifying this problem in the training data? How is the person finding these biases? And who is making the decision to rectify the issue, and how are they doing it? One may also question whether tech companies should be the ones to be assigned these tasks.
With the significant issues of misinformation and disinformation, we need to understand who the gatekeepers are and whether they are the appropriate entities to do this.